Artificial Intelligence (AI) and Machine Learning (ML) are at the forefront of technological innovation, driving significant advancements across various industries. These technologies are reshaping how we interact with the world, offering solutions that were once considered science fiction. This article explores the fundamentals of AI and ML, their applications, benefits, challenges, and future potential.
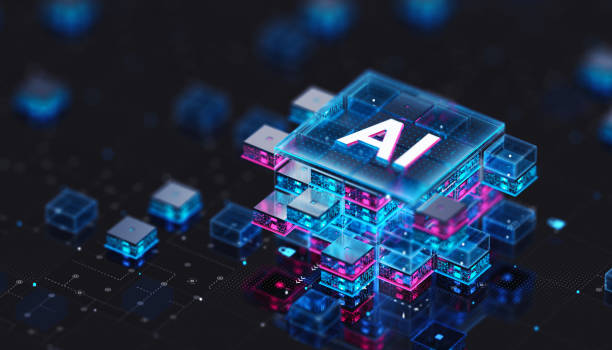
Understanding Artificial Intelligence and Machine Learning
Artificial Intelligence (AI) refers to the simulation of human intelligence in machines that are programmed to think and learn. AI encompasses a wide range of technologies, from simple rule-based systems to sophisticated neural networks that mimic human cognitive processes. AI can be categorized into two types:
- Narrow AI: Also known as Weak AI, this type is designed to perform specific tasks, such as virtual assistants or recommendation systems. Narrow AI operates within a limited scope and does not possess general intelligence.
- General AI: Also known as Strong AI, this type aims to possess human-like cognitive abilities, including reasoning, problem-solving, and understanding. General AI remains theoretical and has not yet been achieved.
Machine Learning (ML) is a subset of AI that focuses on the development of algorithms that enable machines to learn from data and improve their performance over time without being explicitly programmed. ML algorithms identify patterns, make predictions, and adapt to new information. Key types of ML include:
- Supervised Learning: Involves training models on labeled data, where the input and corresponding output are known. The model learns to predict the output for new, unseen data. Examples include image classification and spam detection.
- Unsupervised Learning: Involves training models on unlabeled data, where the system identifies patterns and structures within the data without predefined outcomes. Examples include clustering and dimensionality reduction.
- Reinforcement Learning: Involves training models through trial and error, where the system learns to make decisions by receiving rewards or penalties based on its actions. Examples include game playing and robotic control.
Applications of AI and ML
- Healthcare: AI and ML are revolutionizing healthcare by improving diagnostics, personalizing treatment plans, and accelerating drug discovery. AI algorithms analyze medical images, predict patient outcomes, and assist in identifying potential health issues at an early stage.
- Finance: In the financial sector, AI and ML are used for fraud detection, algorithmic trading, and risk management. ML models analyze transaction patterns, detect anomalies, and predict market trends, enhancing decision-making and security.
- Retail: AI and ML enhance the retail experience through personalized recommendations, inventory management, and customer service. Recommendation engines suggest products based on user preferences, while AI-driven chatbots provide real-time assistance.
- Transportation: Autonomous vehicles, powered by AI and ML, are transforming transportation by enabling self-driving cars and optimizing logistics. AI algorithms process data from sensors and cameras to navigate roads, improve safety, and reduce traffic congestion.
- Manufacturing: AI and ML improve manufacturing processes through predictive maintenance, quality control, and supply chain optimization. AI systems monitor equipment performance, detect defects, and optimize production schedules to enhance efficiency.
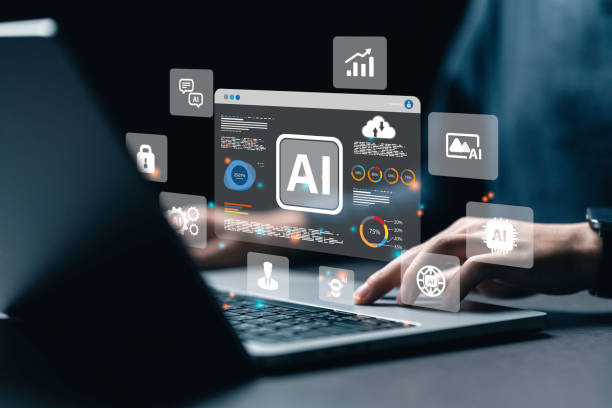
Benefits of AI and ML
- Enhanced Efficiency: AI and ML automate repetitive tasks, streamline processes, and optimize operations, leading to increased productivity and reduced operational costs.
- Improved Decision-Making: By analyzing vast amounts of data and identifying patterns, AI and ML provide valuable insights that support informed decision-making and strategic planning.
- Personalization: AI and ML enable personalized experiences by tailoring recommendations, services, and products to individual preferences and behaviors, enhancing customer satisfaction.
- Innovation: AI and ML drive innovation by enabling the development of new technologies, applications, and solutions that address complex challenges and create new opportunities.
- Scalability: AI and ML systems can scale to handle large volumes of data and adapt to changing conditions, making them suitable for a wide range of applications and industries.
Challenges in AI and ML
- Data Privacy: The use of AI and ML involves collecting and analyzing large amounts of data, raising concerns about data privacy and security. Ensuring that data is handled responsibly and in compliance with regulations is crucial.
- Bias and Fairness: AI and ML algorithms can inadvertently perpetuate biases present in the training data, leading to unfair or discriminatory outcomes. Addressing bias and ensuring fairness in AI systems is an ongoing challenge.
- Explainability: Many AI and ML models, especially deep learning systems, are often referred to as “black boxes” due to their complexity. Understanding how these models make decisions and ensuring transparency is important for trust and accountability.
- Job Displacement: The automation of tasks through AI and ML can lead to job displacement and changes in the workforce. Preparing for these changes and providing retraining opportunities is essential for managing the impact on employment.
- Ethical Considerations: The deployment of AI and ML raises ethical questions about the use of technology in areas such as surveillance, autonomous weapons, and decision-making. Establishing ethical guidelines and standards is important for responsible AI development.
Future Trends in AI and ML
- Advancements in Deep Learning: Deep learning, a subset of ML, is expected to continue advancing, leading to more powerful and accurate models for tasks such as image recognition, natural language processing, and speech synthesis.
- AI Ethics and Regulation: As AI and ML become more integrated into society, there will be increased focus on developing ethical frameworks and regulatory standards to ensure responsible and equitable use of these technologies.
- Human-AI Collaboration: The future of AI and ML will involve greater collaboration between humans and machines, with AI augmenting human capabilities and assisting in complex problem-solving and decision-making.
- Edge Computing: Edge computing, which involves processing data closer to the source rather than in centralized data centers, will enable faster and more efficient AI and ML applications, particularly in IoT and autonomous systems.
- AI in Healthcare: Continued advancements in AI and ML will drive innovations in healthcare, including personalized medicine, advanced diagnostics, and improved patient care.
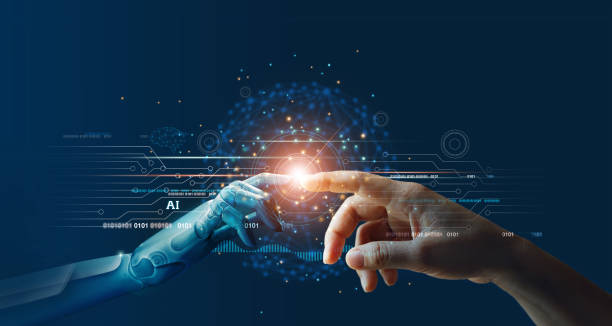
Conclusion
Artificial Intelligence and Machine Learning are transforming industries and shaping the future of technology. By enhancing efficiency, improving decision-making, and driving innovation, these technologies offer significant benefits while also presenting challenges related to data privacy, bias, and ethical considerations. As AI and ML continue to evolve, staying informed about their advancements and implications will be essential for harnessing their potential and addressing the challenges they present. The future of AI and ML promises exciting opportunities and advancements that will shape how we interact with technology and the world around us.